Introduction
In a report prepared in 2014 at the request of the United Nations Secretary-General, there were calls for a data revolution for sustainable development (IEAG, 2014). Data is the lifeblood of decision-making and satellite earth observation could offer novel capabilities for measuring and monitoring socioeconomic conditions (Hargreaves & Watmough, 2021). New technologies are leading to exponential growth in the volume and types of data available (IEAG, 2014), and satellite imagery along with artificial intelligence (AI) – powered machine learning (ML) tools offer intriguing potential for sustainable development (Burke et al., 2021).
There is a new gold rush underway – a big data rush (MacFeely, 2019), where bigger, faster and more detailed data is now available than ever before. Big data has been defined by the UN Statistical Commission (2018, p. 4) as ” any data sources that can be described as; high volume, velocity and variety of data that demand cost-effective, innovative forms of processing for enhanced insight and decision making”. Satellite imagery big data fits this description, and as it becomes more accessible to academics, governments, companies, non-government organizations (NGOs), civil society organizations, and individuals, the possibilities for its use are endless. Satellite images can be analyzed for a wide range of purposes that can include measuring poverty across the global south (Hargreaves & Watmough, 2021; Jean et al., 2016), agricultural productivity (Murugan et al., 2017), water supply and sanitation monitoring (Bayissa et al., 2019; Mori et al., 2011), rural development (Hargreaves & Watmough, 2021), and infrastructure development (Burke et al., 2021), but to name a few. Satellite imagery can be used to measure performance against targets set by the Sustainable Development Goals (SDGs) (IEAG, 2014). Big data allows governments and international institutions make informed decisions that are in the best interests of locals, nations, and the global population.
This report will look several examples of successful use of big data from satellite imagery. There will then be a discussion on the limitations and challenges in the use of big data from satellite imagery, and the true complexities involved in its use. To conclude with there will be some final thoughts on big data from satellite imagery and its place in future decision-making processes.
Poverty and Rural Development
If the international community is to achieve the SDGs it needs appropriate data to measure rural poverty and development, that can inform policies to alleviate poverty (Castañeda et al., 2018). Satellite imagery data can augment traditional survey data collection and generate more comprehensive diagnostics of rural poverty and development in low- and middle-income countries (LMICs) (Hargreaves & Watmough, 2021). New tools such as satellite technology can enable the detection and visualization of economic and social dimensions of rural spaces, by integrating the resulting data with data collected by more traditional methods (Fritz et al., 2019). Jean et al., (2016) used a combination of survey and satellite data from five African countries to show how a convolutional neural network (CNN), or a network architecture for deep learning that learns directly from data, can be trained to identify image features that can explain local-level economic variations. Please view the video by Neal Jean explaining this process.
Satellite derived earth observation data can thus be used to estimate different dimensions of poverty and socio-economic conditions and support data derived from census and surveys (Hargreaves & Watmough, 2021). Natural and artificial landscape features can be detected from space that are commonly associated with human wellbeing (Watmough et al., 2013a, 2013b). Comparisons between nighttime imagery that indicate rate of electrification, and signifiers from daytime imagery such as building locations and size, type of roof, road infrastructure and material, number of cars, and type of farmland, are used in Sri Lanka to estimate and predict poverty rates at local levels using deep learning-based CNN (Engstrom et al., 2022).
Ayush et al., (2020) uses consumption expenditure determined from high resolution satellite imagery to predict poverty at village level in Uganda, where high-quality ground data exists as a benchmark. Figure 1 illustrates classification of ground level objects, including buildings and vehicles, which are used to determine poverty levels.
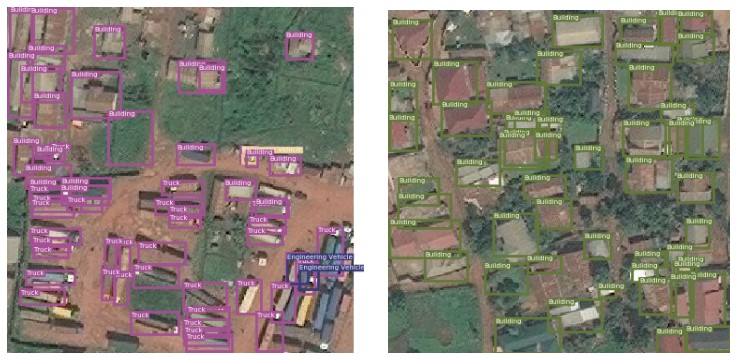
Source: Ayush et al (2020, p. 4414)
Satellite imagery big data can facilitate geographically targeted poverty interventions, once results are validated by comparison with data collected by traditional methods, and expert and local knowledge is incorporated (Hargreaves & Watmough, 2021).
Agricultural Productivity
Drought events affecting agricultural productivity are becoming increasingly frequent and early warning indicators are becoming critical to mitigating economic and social impacts. Agricultural drought in Spain in 2005 led to a 40% reduction in cereal production and non-irrigated crop and pasture losses were estimated to be 2500 million euros (Sepulcre-Canto et al., 2012). Drought conditions are expected to worsen in the future due to climate change making them more frequent and widespread (Gomez-Gomez et al., 2022). Rainfed agriculture is sensitive to seasonal climate variability and integrating multiple variables representing different climatic and agricultural components may offer more robust agricultural drought and early warning system (Sepulcre-Canto et al., 2012). A recent case study completed for Ethiopia by Bayissa et al (2019) showed that a combined drought indicator, using satellite agrometeorological input parameters, could be used to develop an agricultural drought and early warning system. Figure 2 highlights the difference between 2006 when there were wet conditions observed in many areas of Ethiopia, and 2009 plus 2015 when there were severe drought conditions observed in many areas.
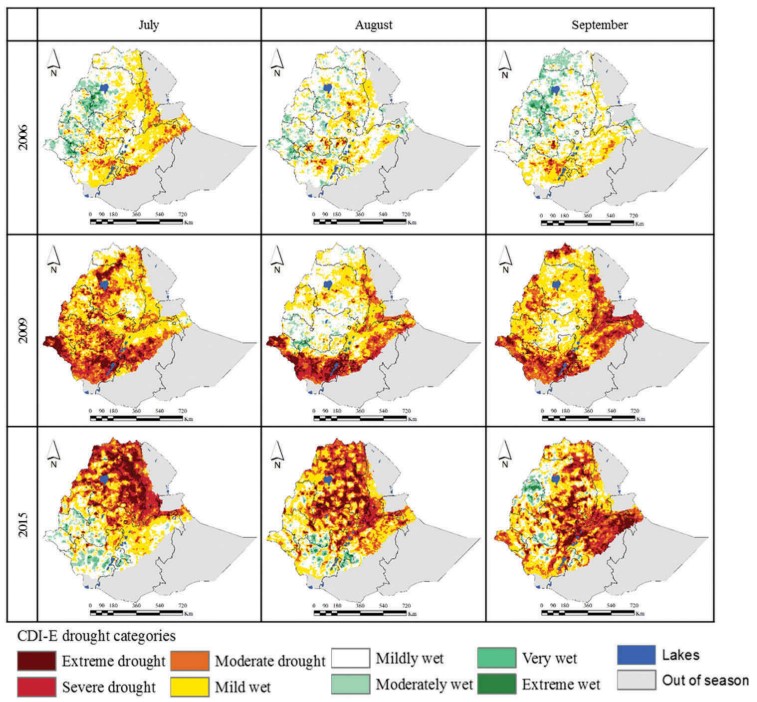
Source: Bayissa et al., (2019, p. 16)
As the failure of seasonal rainfall is often the precursor for agricultural drought in Ethiopia, the CDI-E can be used in developing a drought forecasting model to enhance preparedness and mitigate associated socioeconomic impacts (Bayissa et al., 2019).
Sustainable Development Goals (SDGs)
The global development community are working tirelessly to help countries achieve their SDG targets. However, there needs to be a way to measure progress, and maximize the benefits from technological advances, to ensure no country gets left behind. The 17 SDGs have 169 targets, and these are measured using 232 indicators, but as of May 2018 less than half (40%) of the SDG indicators can be populated due to lack of available data (MacFeely, 2019). Satellite imagery is a key method of gathering this data and has the potential to fill this data gap, as satellites constantly orbit the Earth and quality, costs, and accessibility continue to improve.
Sustainable development outcomes such as eliminating hunger, promoting health and well-being, and building sustainable communities (Burke et al., 2021) can be measured with help from satellite imagery. As far back as 2014, satellite data was being collected and used in Cote d’Ivoire and Senegal to measure progress against the SDGs reliably and cheaply (IEAG, 2014). There is great potential for growth in satellite imagery data collected in this area in the future.
Humanitarian Applications
We live in an increasingly complex world defined by persistent crises. New technologies such as satellite imagery allow for the collection of enormous amounts of increasingly accurate data that can be use in the search for solutions to humanitarian crises. Satellite imagery allows for estimates of probability of drought and famines by calculating variables such as crop yield, temperature, humidity, atmospheric pressure, and rainfall, amongst many other factors (Guida, 2021). Anomalous sea-surface temperatures on the El Nino-Southern Oscillation, which influences precipitation across the tropics and subtropics, can have important value as part of the observation of key factors impending drought (ESA, 2015). In Guinea, the NGO Doctors Without Borders used satellite imagery to map 90000 buildings over a period of 5 days using 244 volunteers, as they were missing from local maps, to ensure aid in the fight against the spread of the Ebola virus (Froment, 2020). Figure 3 illustrates map improvements based on satellite imagery to help with a measles vaccination campaign in Malawi.
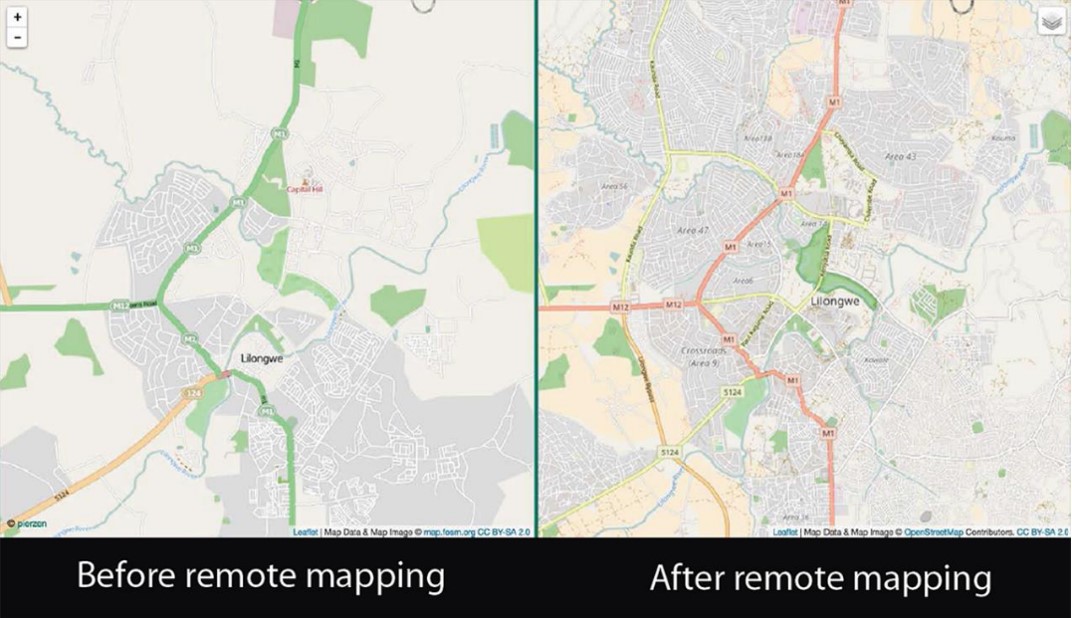
Source: Froment, (2020, p.14)
Satellite imagery has been used to monitor wildfires from above, both in terms of intensity and extent of large-scale fires, to pre-empt danger to populated areas and ensure people are evacuated (Guida, 2021). Will Marshall, CEO of Planet, explain in the following video the benefits of satellites in agriculture, monitoring wildfires, and updating maps.
Figure 4 shows wildfires in Australia in 2019 that burned 18.6m hectares of land and 3500 homes (Froment, 2020), captured by satellites from above. Images such as this can help with the emergency response by providing information that helps with installing precautionary measures and reducing response times (Froment, 2020).
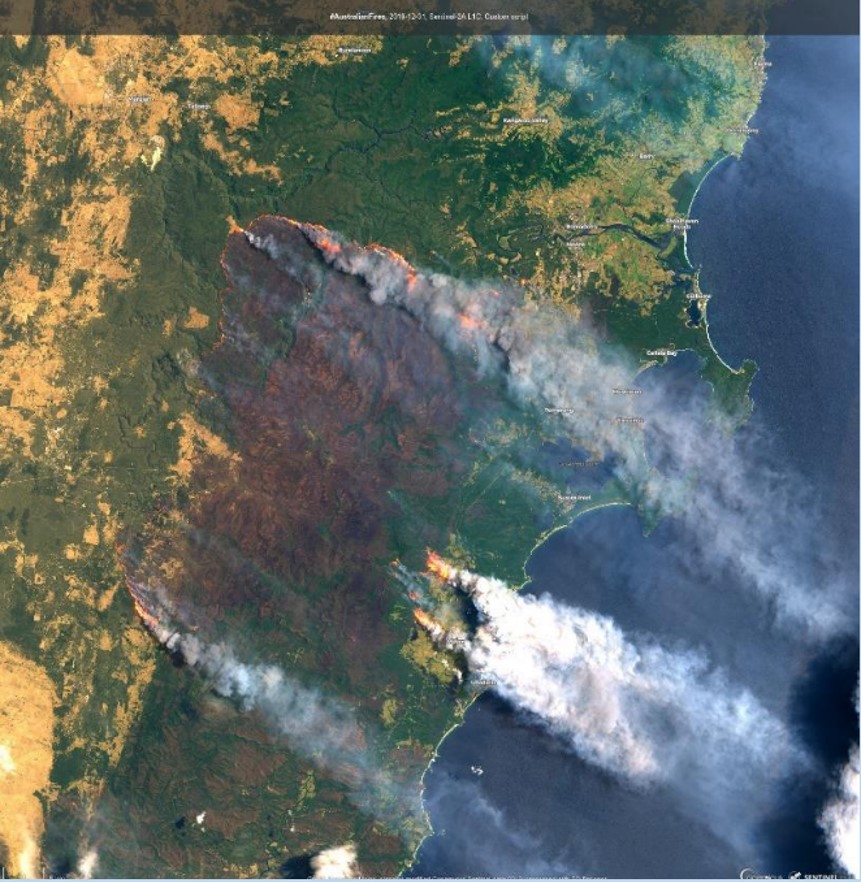
Source: Sentinel2 Copernicus EMS (Froment, 2020, p. 13)
The flow of migrants across the Mediterranean can be monitored using satellite imagery by remotely detecting and tracking vessels, so that the relevant authorities can be informed, or warnings can be issued to search and rescue in case of danger (Guida, 2021). As satellites numbers increase, and accuracy and accessibility improve, the potential applications for this technology in humanitarian efforts will also increase.
Limitations and Challenges
Big data requires complex artificial intelligence (AI) algorithms and machine learning methods to make sense of it (Hargreaves & Watmough, 2021). Satellite-based machine learning models are only as good as the data on which they are trained and securing access to high quality training data in development contexts can be challenging (Burke et al., 2021). There is also the risk of over-reliance on technology and inadequate testing of resulting data before its use in development decision making (Blumenstock, 2018).
There is a concerted push towards the uptake of big data for the SDGs (MacFeely, 2019). Care must be taken to ensure that subnational, local scale heterogeneities, and the agency of the local poor themselves are paramount, when diverse countries are amalgamated with a minimal suite of satellite imagery proxies that predict a limited set of socioeconomic indicators (Hargreaves & Watmough, 2021). There is a need to transfer earth observation capabilities using satellite technology to end users including National Statistics Offices (NSOs) and National Mapping Agencies (NMAs) to improve reporting and deliver informed policy at the sub-national level (Kavvada et al., 2020).
Satellite imagery collected is of varying resolutions, and thus of varying quality. Optical satellites are often hindered by cloud cover and are often not of high enough resolution to detect important ground details (Froment, 2020). Synthetic Aperture Radar (SAR) technology can penetrate cloud cover and is available at higher resolutions than optical satellite imagery, but it is also more expensive. SAR technology is particularly useful in disaster prevention and emergency response activities (ESA, 2015).
Big data from satellite imagery presents statistical and governance challenges and pitfalls that can be legal, ethical, technical or reputational in nature (MacFeely, 2019). IT infrastructure needs to improve in terms of resilience and security, if it is to manage large amounts of data and ensure end-user confidence and trust (IEAG, 2014).
There is the potential danger of ignoring the unintended consequences of satellite imagery, in favor of the potential of this revolutionary technology (Lichtman & Nair, 2015). The power to derive value from big data tends to rest in the hands of the few who have access to big data and the technology to analyze and interpret it (Blumenstock, 2018). Algorithms designed to make sense of big data need to be studied, reviewed and vetted by experts to eliminate inherent bias and unfairness, and ensure they are fit for purpose (IEAG, 2014). Solutions derived from satellite imagery big data can often work for those that are already empowered rather than the vulnerable populations they are intended for (Blumenstock, 2018).
Conclusions
There is an urgent need to mobilize the data revolution for all people (IEAG, 2014). Data needs to be accessible to all and much work needs to be done to ensure that increased data reduces rather than increases inequalities. As MacFeely (2019) explains, digital and data divides exist as a result of access barriers such as social, gender, geographic or economic, which can lead to exclusion.
Satellite imagery big data has great potential in development and humanitarian contexts, but it must be approached with caution. While the datafication of life is destined to continue, it needs to work for the betterment of humanity. There needs to be collaboration with locals, accountability in decision making, validation of data findings, and trust in the findings.
Big data does not automatically imply better data and more data does not always lead to more insight (MacFeely, 2019). Whether big data is a friend or foe remains to be seen. The proof will be evident from the benefits gained from its collection and use over time.
Above all it must be remembered that we are conducting research about people, and for people, and there is a moral imperative to remember this (Hargreaves & Watmough, 2021).
Reflections
This blog exercise has been both challenging and rewarding. It has given me the opportunity to examine satellite imagery big data more closely which is something I will be working closely with for development purposes. While there are benefits from satellite imagery big data in development and humanitarian contexts, there are also major concerns, limitations, and ethical considerations that need careful thought.
Constructing and managing the blog has increased my appreciation for its potential and value in ‘Communication for Development’. Teamwork has been an essential component of our success in constructing the blog and working across four time-zones with a seventeen-hour time difference. Communications channels such as WhatsApp and University Canvas have enabled continuous discussions and communications which has been critical to sharing the workload and ensuring we meet deadlines. Assigning blog roles to each team member had the effect of creating a team of five motivated leaders, but in different areas of the assignment, to ensure we arrived at a user friendly and smart blog interface, with interesting and captivating blogposts.
Increasingly communications happen through some form of digital platform and writing a blog that links to Twitter and LinkedIn has been quite appropriate for ‘Communication for Development’. While Millennials got to see social media and big data technology evolve, generation Zs were born immersed in social media and big data technology. Big data from satellite imagery is yet another way of increasing the ‘datafication’ of work/life and fits well within the theme of ‘Social Media, Datafication and Development’. Whether you are anti big data or pro big data, it is here to stay, and so is the datafication of work/life. Questions surrounding its collection, analysis, storage, and influence on decision making, need asking more frequently and more fervently to ensure we maximize its value in development and humanitarian contexts.
References
Ayush, K., Uzkent, B., Burke, M., Lobell, D., & Ermon, S. (2020). Generating interpretable poverty maps using object detection in satellite images. IJCAI International Joint Conference on Artificial Intelligence, 2021–Janua, 4410–4416.
Bayissa, Y. A., Tadesse, T., Svoboda, M., Wardlow, B., Poulsen, C., Swigart, J., & Van Andel, S. J. (2019). Developing a satellite-based combined drought indicator to monitor agricultural drought: a case study for Ethiopia. GIScience and Remote Sensing, 56(5), 718–748.
Blumenstock, J. (2018). Don’t forget people in the use of big data for development. Nature, 561, 170–172.
Burke, M., Driscoll, A., Lobell, D. B., & Ermon, S. (2021). Using satellite imagery to understand and promote sustainable development. Science, 371(6535), 1–6.
Castañeda, A., Doan, D., Newhouse, D., Nguyen, M. C., Uematsu, H., & Azevedo, J. P. (2018). A New Profile of the Global Poor. World Development, 101, 250–267.
Engstrom, R., Hersh, J., & Newhouse, D. (2022). Poverty from Space: Using High Resolution Satellite Imagery for Estimating Economic Well-being. World Bank Economic Review, 36(2), 382–412.
ESA. (2015). Satellite Earth observations in support of climate information challenges. CEOS Earth Observation Handbook for COP21, 59.
Fritz, S., See, L., Carlson, T., Haklay, M. (Muki), Oliver, J. L., Fraisl, D., Mondardini, R., Brocklehurst, M., Shanley, L. A., Schade, S., Wehn, U., Abrate, T., Anstee, J., Arnold, S., Billot, M., Campbell, J., Espey, J., Gold, M., Hager, G., … West, S. (2019). Citizen science and the United Nations Sustainable Development Goals. Nature Sustainability, 2(10), 922–930.
Froment, R. (2020). Use of Earth Observation Satellites to improve effectiveness of humanitarian operations.
Gomez-Gomez, J. de D., Pulido-Velazquez, D., Collados-Lara, A. J., & Fernandez-Chacon, F. (2022). The impact of climate change scenarios on droughts and their propagation in an arid Mediterranean basin. A useful approach for planning adaptation strategies. Science of the Total Environment, 820, 153128.
Guida, E. (2021). The use of satellites in humanitarian contexts (Issue November).
Hargreaves, P. K., & Watmough, G. R. (2021). Satellite Earth observation to support sustainable rural development. International Journal of Applied Earth Observation and Geoinformation, 103(March), 1–13.
IEAG. (2014). A World that Counts: Mobilizing the Data Revolution for Sustainable Development. In Trusted Data: A New Framework for Identity and Data Sharing (pp. 323–370).
Jean, N., Burke, M., Xie, M., Davis, W. M., Lobell, D. B., & Ermon, S. (2016). Combining satellite imagery and machine learning to predict poverty. Science, 353(6301), 790–794.
Kavvada, A., Metternicht, G., Kerblat, F., Mudau, N., Haldorson, M., Laldaparsad, S., Friedl, L., Held, A., & Chuvieco, E. (2020). Towards delivering on the sustainable development goals using earth observations. Remote Sensing of Environment, 247.
Lichtman, A., & Nair, M. (2015). Humanitarian uses of drones and satellite imagery analysis: The promises and perils. AMA Journal of Ethics, 17(10), 931–937.
MacFeely, S. (2019). The Big (data) Bang: Opportunities and Challenges for Compiling SDG Indicators. Global Policy, 10(January), 121–133.
Mori, S., Ihsanullah, Koizumi, A., Maeda, C., Asada, K., Yokota, Y., & Mori, M. (2011). Innovative methodology of demand responsive approach for large-scale water supply and sewerage/on-site sanitation projects in developing countries using participatory GIS with high resolution satellite imagery. Journal of Water Supply: Research and Technology – AQUA, 60(5), 294–304.
Murugan, D., Garg, A., & Singh, D. (2017). Development of an Adaptive Approach for Precision Agriculture Monitoring with Drone and Satellite Data. IEEE Journal of Selected Topics in Applied Earth Observations and Remote Sensing, 10(12), 5322–5328.
Sepulcre-Canto, G., Horion, S., Singleton, A., Carrao, H., & Vogt, J. (2012). Development of a Combined Drought Indicator to detect agricultural drought in Europe. Natural Hazards and Earth System Science, 12(11), 3519–3531.
UN Statistical Commission. (2018). Guidelines on Data Flows and Global Data Reporting for Sustainable Development Goals (Vol. 3).
Watmough, G. R., Atkinson, P. M., & Hutton, C. W. (2013a). Exploring the links between census and environment using remotely sensed satellite sensor imagery. Journal of Land Use Science, 8(3), 284–303.
Watmough, G. R., Atkinson, P. M., & Hutton, C. W. (2013b). Predicting socioeconomic conditions from satellite sensor data in rural developing countries: A case study using female literacy in Assam, India. Applied Geography, 44, 192–200.