The following lines will explore how algorithms affect how police views and deals with, black communities. Are AI and mathematical algorithms being used by police departments in an ethical way that promotes equality, or are they being misused and are eventually rooting racism? The discussion will be in light of few arguments from a book that I am currently exploring: Weapons of Math Destruction (2016) by Cathy O’Neil, and an interview with Michelle Alexander, author of The New Jim Crow: Mass Incarceration in the Age of Colorblindness (2010), which is a book that seems I am quite behind on consuming it, which calls for exploring it.
Civil rights and legal scholar, Michelle Alexander presents how the criminal justice system in America is a designed system of racial control. The purpose of this post is to investigate the link between the acclaimed justice system practised by police tactics and AI racist models. Is nowadays AI tools part of this designed system of racial control which Alexander sheds light on? Police are identifying “high-risk areas” through models that are built by mathematical algorithms programmers. This is a heavy complex thread that needs ethical, historical, and technical insights, which are indeed beyond the goal of this launchpad post. But, let us just attempt to unpack this linkage.
Predictive policing
High-Tech surveillance tools are extensively expanding. Whether it is for preventive or monitoring measurements. From extensively distributed security cameras to facial recognition programs and G.P.S. tracking devices. An issue that certainly opens room for dialogues around privacy and the moral investigation of the possibility of witnessing what Alexander names “E-carceration”, and “digital prisons”. With that said, it is one thing to monitor people and a whole another thing to detect “potential” criminals and put a label on them based on assumptions; not on their actual actions. Yes, the 15 years old Minority Report movie became a reality. A reality that seems to be exclusively witnessed in poor communities of colour.
As Ibram X.Kendi, puts it, Alexander’s discussion seems to “[..] struck the spark that would eventually light the fire of Black Lives Matter;” a well-known glorious movement that my colleague Ebba studies in detail in her blog post. One key spark that I would elaborate on is the predictive police models and surveillance technologies that are used to detect “possible” criminals. Young black men are baselessly being labelled as felons due to minimal violations such as lingering, or holding tiny amounts of marijuana (Remnick, 2020); violations that are practised by white people alike without the felony stigma. What is more striking is that sometimes these young men are completely innocent, yet, they are monitored and labelled as “possible felons”, due to their social network. Just because one was unlucky to be born in a poor dangerous neighbourhood, they would have a high probability of developing a criminal record. Criminal records that consequently authorize legal discrimination against these young men all of their lives (Remnick, 2020). How will this cycle break? How can a young man categorized as a possible criminal be able to find a job? Open a bank account? or progress in his life?
An Example
In her book, Weapons of Math Destruction, O’Neil (2016, chapter 5) demonstrates an example of unfairness that resulted from one police predictive model developed by Chicago Police Department and funded by the National Institute of Justice. They established a list of around 400 people that are predicted to commit criminalities. These people were also ranked according to their tendency to take part in homicides. The police aim was to knock on these men’s doors and “warn” them that they are being monitored and to think twice before committing a crime. These models are designed by the logic that “Birds of a feather, statistically speaking, do fly together”, O’Neil (2016, p.87). One of the people who received this door knock is a 22-year-old man named Robert McDaniel. McDaniel who had never been charged with any gun violation or any other crime, as he later informed Chicago Trune, received a warning from the police and a message to “watch out”. O’Neil pictures, what would happen to this man if in the future he falls into a foolish violation like a barroom fight, for instance. Foolish violations that we are all equally vulnerable to fall into. Yet, in the case of this young man, such incident would lead to the following: “the full force of the law will fall down on him, and probably much harder than it would on most of us. After all, he’s been warned,[emphasis added]”, as O’Neil rightly pictures it. I would also argue, how would these young men have the incentive for prosperous habit formation and positive behaviour change under such treatment and labelling? Labelling that is a product of models with many biased assumptions.
Is AI racist?
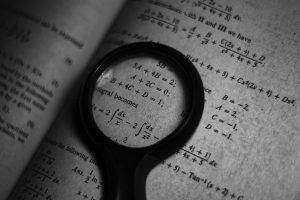
“Our own values and desires influence our choices; from the data, we choose to collect to the questions we ask. Models are opinions embedded in mathematics.” O’Neil (2016, P.27).
O’Neil (2016) explores how models are never bias-free, which is something predictable from the perspective of mathematical and statistical limitations. At the end of the day, we have lots of variables, assumptions, and objectives when designing predictive models. It is anticipated that these models have blind spots, hence, there is always room for updating and fixing, and that is why we cannot completely rely on them. Yet, as, O’Neil argues “A model’s blind spots reflect the judgments and priorities of its creators” (p.26). “Is AI racist?” is an ironic question. AI is not racist obviously; I mean yes, it is, if the creators are! (consciously or subconsciously). Here comes in Jannie Jackson key reminder that we shall be aware of a narrow understanding of racism. She highlights the necessity to zoom out and look at the big picture of patterned systematic racism and to understand “patterned behaviours, the institutional, the legal, the policy level forms of racism, that don’t reply on malicious intent.”, she adds “Of course, oftentimes, that is still in the mix for many things” (Jackson,2019). Hence; when designing models, O’Neil invites us to “[..] explicitly embed better values into our algorithms, creating Big Data models that follow our ethical lead” (p.162).
How unsettling it would be when the problem of unfairness (whether it is due to racism or unintentional biases and blind spots) cannot be properly tracked and analysed? How concerning is it when data-driven logic dominates the policing and law enforcement services? It opens the concern of covering hidden racism under the “data and numbers don’t lie” claim. If we assume, we can hold a police officer accountable, how can we hold AI models accountable? Who participates in these predictive models? What software do these models rely on? The base generator of AI services is becoming a “black box” as O’Neil (2016) rightly puts it.
let’s shift away from thinking about risky individuals to how risk is produced by our institutions, by our policies, by our laws.
Jannie Jackson
From ComDev’s lens, once again, we are witnessing an oversimplified discourse around pressuring social, economic, and human problems. Discourses that wipe away two key structural words: Politics and Ideology. Here I ponder, how will the AI architecture look like if the designers, (computer scientists, coders, statisticians) were working hand in hand with socialists, anthropologists, scholars, historian, psychologists … etc, and above all: the people? People of the targeted built models? Models that are private and the public has no accesses to it; although these models are used to determine many people lives.
Cracks
It is not merely a concern of individual cases of witnessed racism by some police officers, or some unintentional blind spots in established monitoring models. It is the crack in the holistic logic and reasoning of addressing these systematic problems by providing superficial solutions and the liquefaction of the power, ideology, and race discourse. A crack that is finding its way to AI and predictive models. Speaking of cracks, I reckon Leonard Cohen’s words: “There’s a crack in everything. That’s how the light gets in”.
What are your thoughts about this? would love to read your insights and comments!
References
- Jackson, J. (2019). ‘Black Communities Are Already Living in a Tech Dystopia’.Retrieved October 10, 2020, from https://fair.org/home/black-communities-are-already-living-in-a-tech-dystopia/
- O’Neil, C. (2016). Weapons of math destruction: How big data increases inequality and threatens democracy (First edition ed., Vol. 4.1). New York, NY, United States: Broadway Books. doi: http://governance40.com/wp-content/uploads/2019/03/Weapons-of-Math-Destruction-Cathy-ONeil.pdf
- Remnick, D. (2020). Ten Years After “The New Jim Crow”. The New Yorker. Retrieved October 10, 2020, from https://www.newyorker.com/news/the-new-yorker-interview/ten-years-after-the-new-jim-crow
Other insightful related links
- “Everything and nothing have changed”, Michele Alexander reflects on the10th anniversary of the New Jim Crow book https://www.nytimes.com/2020/01/17/opinion/sunday/michelle-alexander-new-jim-crow.html
- “How AI can reinforce biases in the criminal justice system”, overview video by CNBC news https://www.youtube.com/watch?v=ZMsSc_utZ40
- America’s police: an eager customer for Amazon high tech surveillance tools https://www.nbcnews.com/tech/security/amazon-developing-high-tech-surveillance-tools-eager-customer-america-s-n1038426
- Reflection on “The broken-Windows” theory https://www.npr.org/2016/11/01/500104506/broken-windows-policing-and-the-origins-of-stop-and-frisk-and-how-it-went-wrong?t=1602237208742
- A conference on Government Surveillance and Race https://www.c-span.org/video/?407901-1/discussion-racial-bias-government